Since someone made the mistake of giving me a soap box, I might as well use it!
*Climbs on soapbox, grabs bullhorn*
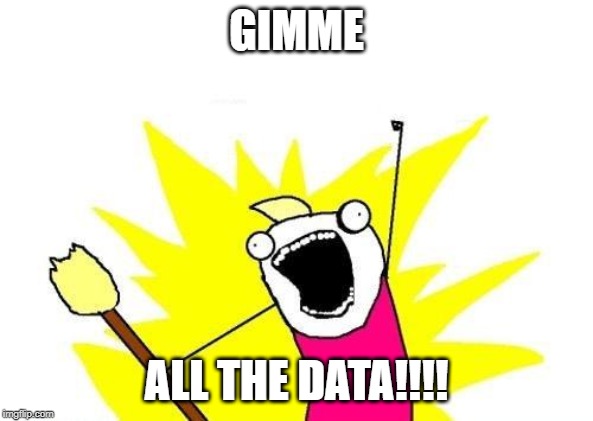
Well, not really. That’s it. That’s the post. 🙂
So, data is the talk of the town, especially in the context of the p-word that shall not be fully named in my presence. And what I am currently hearing from the higher places is pretty much what’s in the above meme.
So, we need to think critically about data. After all, if we’re going to demand and assess critical thinking from our students, the least we can do is turn that spotlight on our own practices, no?
There have been several really good books on the subject of critically thinking about data, focusing on different aspects in which data use has been problematic and how, if we are going to expand our data collection, analysis, and interpretation, we had better be extremely careful about how we go about it. And when it comes to this, there is no salvation by software. No amount of statistical sophistication can make up for the issues discussed in these books.
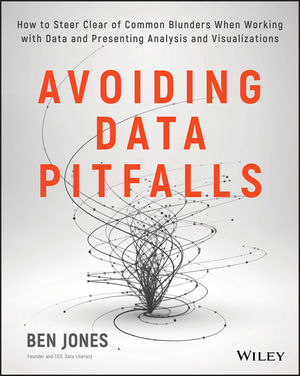
This is not necessarily about getting the math right, although that is important and indispensable. And if this is something you guys are interested in, I would highly recommend Ben Jones’s Avoiding Data Pitfalls: How to Steer Clear of Common Blunders When Working with Data and Presenting Analysis and Visualizations (sorry, publisher’s link since we don’t have it in our Library).
Jones identifies seven potential pitfalls, and yes, some involve getting the math wrong. He also conveniently provides a checklist in the last chapter, for analysts (aren’t we all, at this point?) to determine whether they have fallen into said pitfalls.
Just briefly, here are the seven pitfalls (take a second to appreciate the alliterations):
- epistemic errors – how we think about data: for instance, assuming that the data is a perfect reflection of reality, or using data to verify a previously-held belief;
- technical traps in how we process the data: especially likely if we are merging data from different sources and end up with a ton of “dirty data”, missing data, mismatched or redundant data, or mixing up different units of measurement;
- mathematical miscues, which I less poetically called getting the math wrong;
- statistical slipups: again, there is no salvation by software. No amount of fancy statistical footwork can save us from errors and misinterpretation;
- analytical aberrations: missing signals or its opposite, treating the noise as signal, drawing conclusions not supported by our statistical tests, using metrics that don’t matter;
- graphical gaffes: my rule: #nopiecharts. But worse, one can mislead with data visualizations;
- design dangers: bad design.
What I want to focus on here are a few ideas to think critically about data. I’m not smart enough for a quick and easy checklist but I got sources.
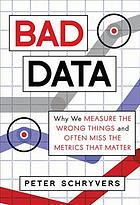
One of the best books on cautionary tales when it comes to uncritically embracing data is Peter Schryvers’s Bad Data: Why We Measure the Wrong Things and Often Miss Metrics that Matter (COD Library link).
[I should note though, that Schryvers uses the now-debunked John Bargh’s study on priming without mentioning said debunking (nicely explained here by Andrew Gelman). That’s a bit disappointing.]
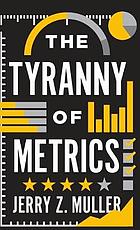
In his book, The Tyranny of Metrics (COD Library link), historian Jerry Z. Muller writes much more in the style of a polemicist, albeit supporting his points with multiple examples, some overlapping with Shryvers’ examples (standardized tests, Compstat, etc.). But, as mentioned, he is much more scathing:
“There are things that can be measured. There are things that are worth measuring. But what can be measured is not always what is worth measuring; what gets measured may have no relationship to what we really want to know. The costs of measuring may be greater than the benefits. The things that get measured may draw effort away from the things we really care about. And measurement may provide us with distorted knowledge—knowledge that seems solid but is actually deceptive.
Muller, Jerry Z.. The Tyranny of Metrics (p. 3). Princeton University Press. Kindle Edition.
Although they cover some of the same grounds, the key difference between them is that Schryvers thinks metrics can be done right, but it’s not easy. Muller has no such compunctions. I would reconcile these two points by saying that if we were to do metrics “right”, then, the people demanding them would demand other newer, shinier metrics, reproducing the pitfalls of the previously used metrics: simplicity, presumed objectivity and completeness, and limited attention to complexity, validity, or reliability. Muller sees the fixation on metrics as a tool of power from people who do not trust professional judgment or experience and therefore prefer to substitute a neatly quantifiable number. Muller considers then the fixation on metrics (and the never-ending demand for more data from which to derive metrics) as a political project (which, you know, it kinda is), what he called the “uncritical adoption of metric ideology“.
For Muller, the problem is not so much metrics as much as metric fixation:
- “the belief that it is possible and desirable to replace judgment, acquired by personal experience and talent, with numerical indicators of comparative performance based upon standardized data (metrics);
- the belief that making such metrics public (transparent) assures that institutions are actually carrying out their purposes (accountability);
- the belief that the best way to motivate people within these organizations is by attaching rewards and penalties to their measured performance, rewards that are either monetary (pay-for-performance) or reputational (rankings).”
Muller, Jerry Z.. The Tyranny of Metrics (p. 18). Princeton University Press. Kindle Edition.
Both authors also zero in on some key general issues:
the well-known dictum that “not everything that can be counted counts, and not everything that counts can be counted.”
And then, one I have been obsessing about for a long time: Goodhart’s Law which can be paraphrased as “when a measure becomes a target, it ceases to be a good measure.” Not only that but such measures, once they become targets, are bound to gamed. In both books examples of Goodhart’s Law abound, from cheating on standardized tests, to Compstat, to body count stats during the Vietnam war, and many more.
A focus on metric, usually single and simple one, is also bound to stifle innovation and creativity because who wants to take risks when you know this might hurt your ranking on the metric. Better stick to what safely works. But such a fixation also encourages a focus on short-term, easily measurable results rather than more structural, long-term projects where the benefits might not materialize for a while.
Part of the problem is a tendency, once it is agreed that something (anything!) has to be measured, is to measure what is easily measurable and use that as the metric of reference, something that is especially problematic when the outcomes are complex and multifaceted. In addition, once a simplistic measure has been selected, standardizing it (so it has broad application irrespective of qualitative differences) results in even further loss of information.
Anyone who has engaged in evaluation research is familiar with the concepts of input, activities, output, and outcomes. Both authors note that it is common to use inputs and outputs as measures when, in fact, we are interested in, and should focus on, outcomes.
Even more concerning, for both authors. is what happens when Goodhart’s Law settles once a metric has become a target:
- gaming through “creaming”: if a school will be evaluated by its pupils’ test scores, it might predominantly select high-performing pupils. A surgeon might elect to do only “easy” surgeries rather than high-risk ones where the outcomes are more likely to not be good.
- Lowering standards: that one is pretty much self-explanatory. Grade inflation might go into that.
- Omission or manipulation of data: a lot of that happened in the NYPD during the Compstat days where a lot of data manipulation happened to make the numbers look good or by dissuading people from reporting crimes.
- Straight cheating.
The dilemma is that none of these problems are solved by collecting even more data or reducing complex problems to more simplistic metrics. But once we face metric fixation, it is difficult for people to conceive that maybe, just maybe, you don’t need Big Data but Small Data, and maybe you don’t need more quantitative indices but more qualitative work.
I highly recommend this short video featuring one of my favorite data scientists, Kate Crawford, “Algorithmic Illusions: Hidden Biases of Big Data” and consider how what she talks about applies to how we collect, analyze, and interpret data:
Let’s face it: as mentioned above, part of the impetus for the demand for more metric is a lack of trust in professional judgment and experience. So, there has to be an additional layer of “objective”, “normed” assessment done at a higher level that erases qualitative differences between, for instance, disciplines. Instead, the same objective rubric has to be used. Scores can then be aggregated to deliver an objective and more complete view, even measured by input (“this many papers were assessed”) rather than outcomes. [This is gonna go over really well]
And of course, as part of the p-word initiative, we prepare to engage in the same process with our students in order to capture as much data on them as possible and design as many interventions as we deem necessary, all with good intentions, I’m sure.
But when all is said and done, we should ask ourselves some questions and adopt data mining / metric policies for the following:
The first and most basic question is, of course, what are we trying to do here? What are we trying to accomplish with all the data we collect (or intend to collect)? What is the outcome? Not the input, not the activities or treatment, not the output. Is there only one outcome? Or several?
Do we really need a quantitative measurement for whatever it is we are trying to figure out? Do we really need aggregate measures and a panoramic view, which is the one most given to us by software packages? Would we be better off with qualitative data and close proximity interventions? As Kate Crawford mentions in the video, would we be better served by small data?
Are we sure we are not introducing bias into our data practice?
Is the answer to every question always going to be “we need more data”? Is there a point where this never-ending quest for “more data”, often tied to “let’s buy another analytics software package”, actually ends? Or is it just the easiest answer to every question. It is the least time-consuming. And it is one that falls into one of the myths Kate Crawford highlights: the illusion of completeness and objectivity.
And if we are going to ask students to provide us with more data about them, or if we are going to generate that data through our systems, how strong is our security? How much do we value students privacy (this is the age of Facebook, data is never fully anonymized)? And where do we place the opt-out options? How long do we retain and use student data? Do we ever purge it? Can students request the data we have on them?
After all, isn’t it up to our students to determine whether they want to be subjected to “intrusive advising” (whoever came up with that creepy phrase needs to go away and think about their lives). So, at what point in the process are they asked? And if they say no, what systems do we have in place to not “sweep” their data along with that of the students who agreed to be spied on to intrusive advising?
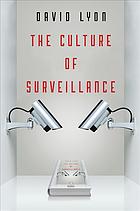
[Putting on my sociologist hat… just kidding, I have it on at all times… I highly recommend David Lyon’s The Culture of Surveillance (COD Library link) on how surveillance technologies have become so individualized, personal, and part of our entire existence that the entire culture has been reshaped to turn us all into both objects of surveillance (think of the many ways COD can keep track of where we are and what we do both physically and online) and surveillance agents ourselves (through social media, communication tech, and other systems).
*Takes a deep breath. Steps down from the soapbox*
Anyway, thanks for reading.
Christine